Vol. 10, No 24, p. 207-231 - 30 abr. 2023
O uso das redes neurais artificiais para obtenção de índices de sustentabilidade de edifícios habitacionais: revisão sistemática da literatura
Carlos Alberto da Costa
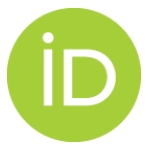
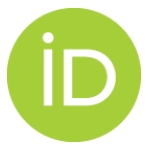
Resumo
A demanda por edifícios mais sustentáveis tem relação com os impactos deste setor, onde a depleção de recursos, incluindo energia, a geração de resíduos e as significativas emissões de gases de efeito estufa, que influem nas mudanças climáticas, dentre outros severos danos, se somam à informalidade e fatores de risco aos operários. As muitas peculiaridades envolvendo os edifícios em seu ciclo de vida pedem métodos de avaliação ponderados, capazes de agregar indicadores para prever o desempenho de diretrizes a eles aplicadas, permitindo a tomada de decisão, em meio à complexidade, sobretudo na construção habitacional. Assim, o objetivo desta pesquisa foi realizar uma revisão sistemática da literatura voltada à aplicação de métodos que se utilizam das Redes Neurais Artificiais para analisar indicadores ambientais, sociais e econômicos; deles obtendo índices de sustentabilidade para os edifícios habitacionais. As abordagens para este fim foram encontradas em 61 de 134 artigos selecionados, porém, a aderência envolvendo índices foi notada somente em oito destes artigos, os quais se apoiaram em poucos indicadores, quase sempre representando diretrizes, ou seja, ações para promover a sustentabilidade. Portanto, verificou-se carência de indicadores da performance produzida por tais diretrizes. Ficou evidente também o desafio de se fazer comparações por meio de um edifício de referência, representante do modo convencional de construção com o qual se está insatisfeito, gerando índices para outros edifícios planejados como sustentáveis.
Palavras-chave
Avaliação de edifícios; Redes neurais artificiais; Indicadores de sustentabilidade;
Índices de sustentabilidade; Revisão sistemática da literatura.
Abstract
The use of artificial neural networks to obtain sustainability indices for housing buildings:
A systematic literature review. The demand for more sustainable buildings is related to the
impacts of this sector, where the depletion of resources, including energy, waste generation and
significant greenhouse gas emissions, which influence climate change, among other severe damages,
add upin formality and risk factors for workers. The many peculiarities involving buildings in
their life cycle, call for weighted evaluation methods, capable of adding indicators to predict
the performance of guidelines applied to them, allowing decision-making in the midst of complexity,
especially in housing construction. Thus, the objective of this research was to carry out a
Systematic Literature Review focused on the application of methods that use Artificial Neural
Networks to analyze environmental, social and economic indicators, obtaining sustainability indices
for housing buildings. Approaches to this end were found in 61 of 134 selected articles, however,
adherence involving indices was noted only in 8 of these articles, which were based on a few
indicators, almost always representing guidelines, that is, actions to promote sustainability.
Therefore, therewas a lack of performance indicators produced by such guidelines. It was also
evident the challenge of making comparisons through a reference building, representative of the
conventional way of construction with which one is dissatisfied, generating indices for other
buildings planned as sustainable.
Keywords
Buildings assessment; Artificial neural networks; Sustainability indicators; Sustainability
indices; Systematic literature review.
DOI
10.21438/rbgas(2023)102415
Texto completo
PDF
Referências
ABNT - Associação Brasileira de Normas Técnicas. NBR 12721: Avaliação de custos unitários
de construção para incorporação imibiliária e outras disposições para condomínios
edifícios. Rio de Janeiro: ABNT, 2006.
Agopyan, V.; John, V. M. O desafio da sustentabilidade na construção civil. São Paulo:
Blucher, 2011. (Série sustentabilidade, 5).
Chen, J. G.; Min, Z. Y. A green construction evaluation model based on BP Neural Networks.
Optimization of Capital Construction, v. 28, n. 5, p. 169-172, 2007.
Costa, C. A. O potencial das redes neurais artificiais como suporte para o desenvolvimento
de índices de sustentabilidade para edifícios habitacionais (ISE-H). Curitiba: Universidade
Tecnológica Federal do Paraná, 2021. (Tese de doutorado).
Ding, Q.; Tong, Q. L.; Tong, H. Q. Evaluation of index for green zoology building and its
definite algorithm in path analysis. Proceeding of the 4th International Conference on
Natural Computation, IEEE Computer Society, Los Alamitos, 2008.
Dong, B.; Cao, C.; Lee, S. E. Applying support vector machines to predict building energy
consumption in tropical region. Energy and Buildings, v. 37, n. 5, p. 545-553, 2005.
https://doi.org/10.1016/j.enbuild.2004.09.009
Foucquier, A.; Robert, S.; Suard, F.; Stéphan, L.; Jay, A. State of the art in building
modelling and energy performances prediction: A review. Renewable and Sustainable
Energy Reviews, v. 23, p. 272-288, 2013. https://doi.org/10.1016/j.rser.2013.03.004
Galvão, M. C. B.; Ricarte, I. L. M. Revisão sistemática da literatura: conceituação, produção
e publicação. LOGEION Filosofia da Informação, v. 6, p. 57-73, 2019. https://doi.org/10.21728/logeion.2019v6n1.p57-73
Hernandez Neto, A.; Fiorelli, F. A. S. Comparison between detailed model simulation and
artificial neural network for forecasting building energy consumption. Energy and
Buildings, v. 40, p. 2169-2176, 2008. https://doi.org/10.1016/j.enbuild.2008.06.013
IEA - International Energy Agence. World energy statistics and balances (Database).
IEA, 2019. Disponível em: <http://www.iea.org/statistics>.
Acesso em: 09 fev. 2021.
JRC - Joint Research Center. Best environmental management practice for the building
and construction sector. Sevilla: JRC, 2012.
Köche, J. C. Fundamentos de metodologia científica: teoria da ciência e
iniciação à pesquisa. Petrópolis: Vozes, 2011.
MPT - Ministério Público do Trabalho; OIT - Organização Internacional do Trabalho.
Observatório de Segurança e Saúde no Trabalho. Promoção do meio ambiente
de trabalho guiada por dados. 2020. Disponível em: <https://smartlabbr.org/sst>.
Acesso em: 09 fev. 2021.
Perez-Lombard, L.; Ortiz, J.; Pout, C. A review on buildings energy consumption information.
Energy and Buildings, v. 40, n. 3, p. 394-398, 2008. https://doi.org/10.1016/j.enbuild.2007.03.007
Qi, B.; Niu, C.; Zhao, J.; Shao, S. Sustainable building evaluation method and its application
based on the BP neural network. Journal of Shenyang Jianzhu University (Social Science),
v. 14, p. 152-156, 2012.
Shama, Z. S.; Motlak, J. B. Indicators for sustainable housing. Materials Science and
Engineering, v. 518, 022009, 2019. https://doi.org/10.1088/1757-899X/518/2/022009
Shi, Q.; Xu, Y.; Chen, S. Green construction alternatives evaluation using GA-BP hybrid
algorithm. Proceeding of International Conference on Management and Service Science,
New Jersey, IEEE Computer Society, 2009.
Si, T. G.; Song, H. P; Liu, J. Y. Artificial neural network in green building assessment.
Journal of Shandong Jianzhu University, v. 23, p. 355-360, 2008.
Silva, V. G. Indicadores de sustentabilidade de edifícios: estado da arte e desafios
para desenvolvimento no Brasil. Ambiente Construído, v. 7, n. 1, p. 47-66, 2007.
UNEP - United Nations Environment Programme. Global guidance for life cycle impact
assessment indicators. Paris: UNEP, 2019. v. 2. Disponível em: <https://www.lifecycleinitiative.org/training-resources/global-guidance-for-life-cycle-impact-assessment-indicators-volume-2/>.
Acesso em: 09 fev. 2021.
Xia, L. M.; Liu, J. J. Research on green building assessment system based on BP Neural
Network and Life Cycle Assessment (LCA). Applied Mechanics and Materials,
v. 357/360, p. 508-514, 2013. https://doi.org/10.4028/www.scientific.net/AMM.357-360.508
Zhang, H.; Shi, X. Economic evaluation of green buildings: A neural network approach.
Proceeding of the International Conference on Information Management, Innovation
Management and Industrial Engineering, Sanya, IEEE Computer Society, v. 2,
p. 327-330, 2012. https://doi.org/10.1109/ICIII.2012.6339845
Zhao, H.; Magoulès, F. A review on the prediction of building energy consumption.
Renewable and Sustainable Energy Reviews, v. 16, p. 3586-3592, 2012.
https://doi.org/10.1016/j.rser.2012.02.049
Zhi, J.Q.; Zhao, J.; Li, N. Green building assessment based on Artificial Neural
Network. Urban Environment & Urban Ecology, v. 23, p. 44-47, 2010.
ISSN 2359-1412